Online Vigilance Analysis Combining Video and Electrooculography Features
Online Vigilance Analysis Combining Video and Electrooculography Features
It is widely acknowledged that one can never emphasize vigilance too much, especially for drivers, policemen and soldiers. Unfortunately, almost every existing vigilance analysis system has its limitations and suffers from poor illumination, horizon of the cameras, together with various appearance and behaviors of the subjects. In this paper, we propose a novel system to analysis vigilance level combining both video and Electrooculography (EOG) features. Our system exploits 16 kinds of features extracted from an infrared camera and 48 kinds of features from horizontal and vertical channels of EOG signals. For one thing, the video features include percentage of closure (PERCLOS), eye blinks, slow eye movement (SEM), rapid eye movement (REM), which are also extracted from EOG signals. For another, other features like yawn frequency, body posture and face orientation are extracted from the video based on Active Shape Model (ASM). The results of our experiments indicate that our approach outperforms that based on either video or EOG merely. In addition, the prediction offered by our model is in close proximity to the actual error rate of the subject. We firmly believe that this method can be widely applied to prevent accidents like fatigued driving in the future.
Publications
Online Vigilance Analysis Combining Video and Electrooculography Features
Videos
Talks
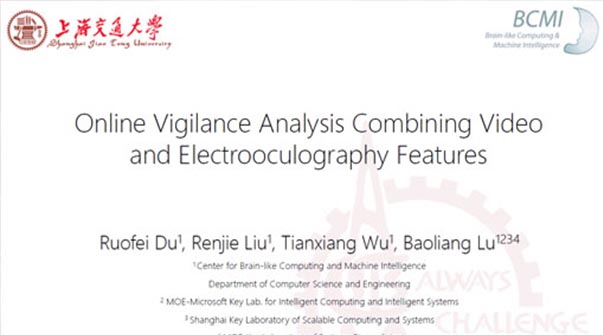
Online Vigilance Analysis Combining Video and Electrooculography Features
Ruofei Du
ICONIP2012, Doha, Qatar.